AI and Historical Language: Shall I Compare Thee to a Human Being?
Artificial intelligence is opening up many avenues for researchers in historical linguistics. For example, you can now programme a bot to write Shakespearean sonnets on demand. Might we soon be able to converse virtually with a speaker from the sixteenth century as well?
Everyone surely knows someone who grumbles about how they are alive at the wrong time. A few years ago, I heard a young man make a melodramatic claim that he would give his right hand to exchange ideas with Immanuel Kant. At the time, despite the usefulness of right hands, it was still possible to say such things: it would not happen anyway.
Today, however, some caution may be warranted when promising body parts in exchange for a conversation with the greatest thinkers and writers of the past. There are more and more projects that try to make this possible with the help of artificial intelligence (AI). In 2019, exactly 350 years after Rembrandt’s death, the Rijksmuseum produced a series of painting lessons narrated in the reconstructed voice of the grandmaster, and a year later there was the miraculous (and unintentionally hilarious) news that British scientists had successfully shown what the voice of a 3,000-year-old mummy would have sounded like (the answer is: eeeeih). And even more astounding is the virtual revival of Einstein ready to answer your questions about his life or science.
Now you can’t simply ask the virtual Einstein anything. If you ask whether he has ever been to the Netherlands, he raises his eyebrows and says that he does not understand the question – the standard response to all questions that his programmers have not prepared. Neither does the virtual Rembrandt independently think of what he should and can say – Dutch linguists from the Dutch Language Institute and Leiden University have done all that for him.
But there is also AI that can generate words and sentences independently, and this too is used by language and literature researchers from Flemish and Dutch research institutes. In response to The Netherlands Reads campaign in 2017, researchers from the Meertens Institute and the University of Antwerp created a literary language robot. The writer Ronald Giphart then set to work with that “robot” to write a new chapter for Isaac Asimov’s science fiction classic I, Robot
with settings that allowed him to do so in the style of Nescio, or Reve and even Dante. And now, in addition to the ‘Asibot’, there is also a ‘Deep-speare’ that writes poems in the style of Shakespeare.
From simple processor to artificial Shakespeare
A computer programme that can write poems appeals to the imagination, of course. But does it really do that all by itself?
Well, not always. In computer programmes that generate language, it is important to distinguish between “rule-based algorithms” and “learning algorithms”. In a rule-based system, in fact, the human programmer is almost completely in charge. For example, to generate a Shakespearean sonnet, the programmer must first assemble a glossary in which she clearly identifies word categories (adjective [A], noun [N], name of month [month] or season [season], verb [V], etc.). And then she has to come up with rules about how those words combine. In order to keep the programming rules reasonably clear in such systems, which are often used for sonnet generator websites, it is a deliberate choice to work from a fairly fixed template, such as the one below, which is based on Shakespeare’s famous ‘Sonnet 18’ (“Shall I compare thee to a summers day”):
List:
A |
N |
month |
season |
V |
yellow dry wooden hot stinky shy |
soup spoon sea pearl butterfly prune |
January February March April May June |
Spring Summer Autumn Winter |
kill tickle bring call grab boil |
Template:
[1] Shall I compare thee to a [A] [N{rhyme_month}]?
[2] Thou art more [A] and more [A{2}].
[3] [A] [N]s do [V] the [A] [N]s of [month],
[4] And [season]’s [N] hath all too [A] a [N{2}].
[5] …
Possible result:
[1] Shall I compare thee to a wooden prune?
[2] Thou art more yellow and more shy.
[3] Dry pearls do tickle the stinky seas of June,
[4] And Winter’s soup hath all too hot a butterfly.
[5] …
In order for lines 1 and 3 to rhyme (as they should according to the ABAB rhyming scheme of the first four lines of Shakespearean sonnets), the programmer will also need to clarify which nouns can be used in the first line of poetry when, for example, the month June is chosen in line 3. So in such cases, the programmer is actually supplying both the recipe and ingredients of the Shakespearean sonnet. The thinking power of the computer programme, on the other hand, can be compared to that of a standard food processor.
The thinking power of the computer programma can be compared to that of a standard food processor
You may call your combi oven AI if you like, but the term is probably a better fit for a system based on “learning algorithms”. Where you have to tell a rules-based system what the ingredients of a cake are and how to put them together, you are basically just telling a learning algorithm: here are some cakes, figure out how to make something like that. And with those relatively minimal instructions, the algorithm then sets to work, looking for patterns that could lead to new cakes, or news stories, or Shakespearean sonnets.
Learning algorithms
The fairly recent emergence of learning algorithms is not only attractive because the programmer has less to decide, but also because it is instructive to explore how the learning algorithm has tried to produce new sonnets, whether successfully or not. At the beginning of the learning process, the AI knows nothing about language – let alone sonnets – except that it is allowed to use letters and spaces. The first proposal for a sonnet by an artificial poet-to-be must therefore be understood as an almost random guess as to which letters and characters can appear in sequence:
s iei een ftr ra nao tnrt tathred nn irt e, ete
e tir i oblteuoe o r ueet tnse ae owe eito hnt eer r r t ree efleeeee t drteei e on thni et,atd eelertsd nittd sssetr stta e oe reaener ont tshi r e bastathoet
yeesb st es n nneen l n
ey ste t emee rhethda t nt oar ift on re e o lt i ta t dt fsthaite o tseai eor e t n oie s pet u etr,nieedseti it s e en t o to t o os t t eeioeotr em ehsbn t hrtiss h i wltteeu nraea ehr th enlsi saof e t lts ney s ferlnh l i
ootin s stws erteno edenh d u dts,t ecoe n ine ie t t h e t esrbee
That first attempt is quite avant-garde, to say the least, but it is nevertheless something of a starting point that can be tested against the examples. The AI keeps making new, more informed attempts. About thirty goes later, the AI seems to have learned that there should only be one space between a series of letters, and more and more letter combinations that resemble real sixteenth-century words are also popping up. Yet the AI still produces a lot of nonsense words, and it hasn’t been able to predict, for example, that the letter i never occurs twice in a row:
on the are thou that the eeartied thoud gipest peare,
the herust me when in the kest mented thee and ast ind pall be sour,
what hee, bith aling and is loveriss thee sich beate the wint,
and there in thee whet thy pinowert in thee thee seest
in thie,
whour the arsell steat still beasten sear thee miines thee the wich hit thees prase
the enesest my chens stall
After sixty attempts, what the AI comes up with is still far from a good sonnet. But still: it has in the meantime grasped that the lines in a sonnet are of equivalent length.
then i all beauty not to breake thee art
thou and he that shave love the sea.
and then i by force me place my fart,
then inother sweet farte thou lost mere
and his fart mace of lies what thou love,
and brace and sear love the arse and doth,
then in thy seas store this thee and hath thee,
and wind in the thou i mate my thing least,
then the lost in the wart of my love faine,
and dare sell me my bear that is my bearty songe
Admittedly, these are not correct sentences and it’s a bit odd that the AI quite often decides ‘fart’ is a good word for a Shakespearean sonnet. But otherwise, its understanding of what a sixteenth-century word could be is quite successful. As if out of nowhere a small piece of Shakespeare has come back to life.
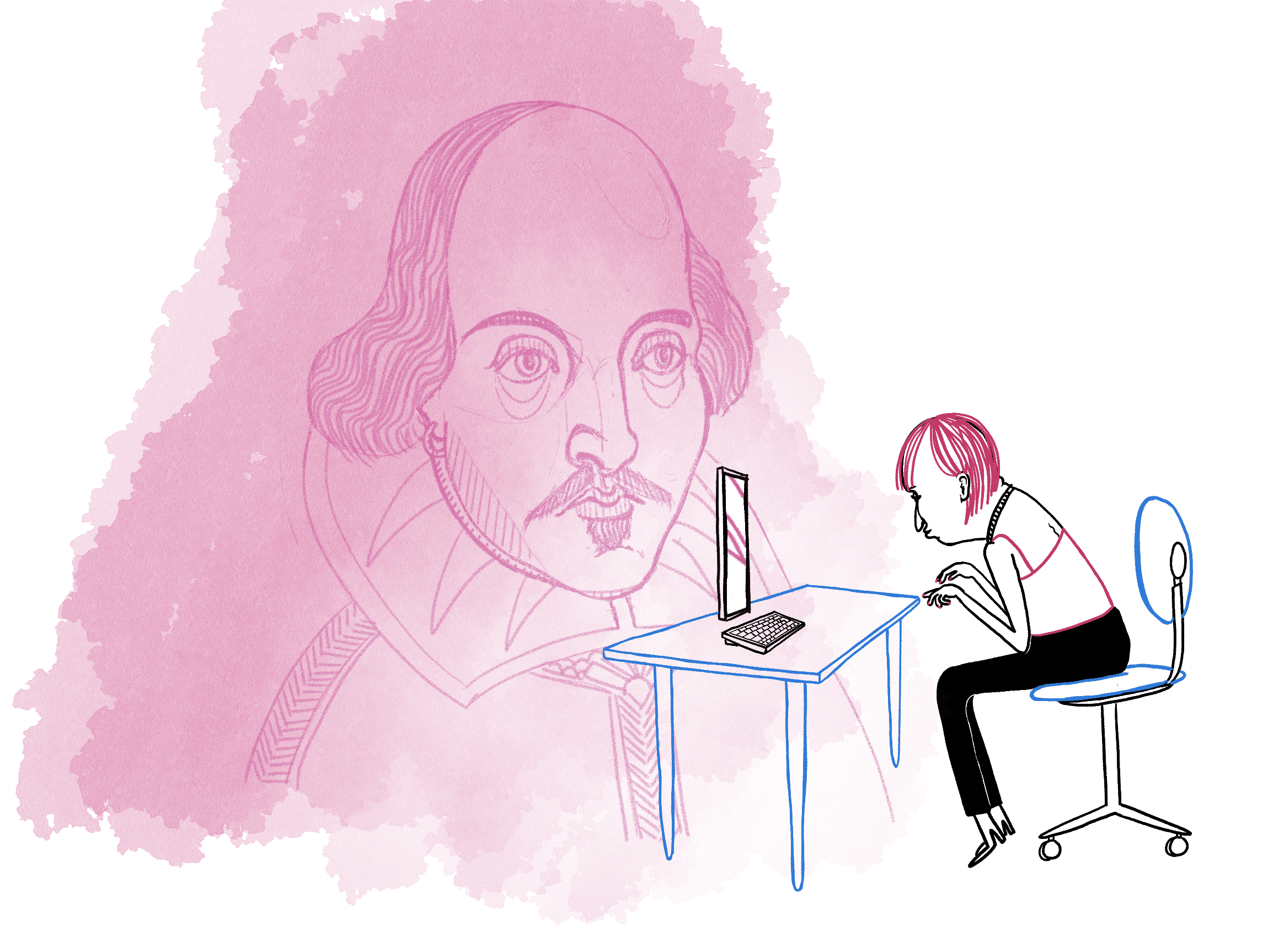
Ⓒ Lauren Fonteyn
AI and historical language research
Letting learning algorithms loose on historical language shows that AI can not only be useful in the present and the future, but that it can also help to unlock the past in a more vivid way. And that is also important for historical text and language research.
Reading a historical text is not only a challenge because older language differs in form from contemporary language. Language users are, in a sense, also programmed to interpret language and texts in a contemporary light. For example, a speaker of contemporary Standard Dutch will be shocked when an elderly woman is suddenly called an “old broad” in a seventeenth-century text that is otherwise very polite – and such differences in meaning can lead to misunderstandings. Even for experienced researchers, it is sometimes difficult and time-consuming to be certain that their contemporary sense of language has not played a role in their analysis.
But a learning algorithm exposed only to seventeenth-century language will work without such contemporary preconceptions and can operate very quickly. Projects are now also being set up at various research institutes (including Leiden University) to enable AI in this way, so that large-scale research into language and texts from the past becomes easier for scientists and the general public.
All’s well that ends well
So for those who feel as though they are living in the wrong century, this is all very positive. But you probably know what I’ll say next: there are still many bumps in the road before we can simulate a real conversation with a speaker from the past. By using AI for historical language research, it becomes clear where AI needs to be improved.
A major drawback of the learning algorithms currently in use is that they require so many examples before they generate anything of value at all. If an AI Twitter account needs tweaking, you only need to wait a day to accumulate 500 million additional examples (the estimated number of tweets sent out into the world per day). Shakespeare, on the other hand, has written only a modest 154 sonnets, and for several centuries he has been unable to produce any more.
Moreover, it is often particularly difficult for AI to make sense of earlier versions of a language, because of the lack of standardised spellings. It might seem easier to write a text if you don’t have to adhere to strict rules, but for learning algorithms, it is essential to have no ambiguity.
However, to get to a system that works, before asking a learning algorithm to emulate Shakespeare’s sonnets, you can expose it to sixteenth-century language more generally. But as we go further back in time, there are fewer and fewer examples of language available, and so for the learning algorithms of today, there is hardly anything to learn.
And then there’s also the issue that, once you start using AI for research purposes, you soon notice that a learning algorithm is still a far cry from a real language user: even the best Shakespeare bot might know it can compare you to a summer’s day, but because of a lack of life experience it really has no idea why.
In short, we are learning more and more about what AI can, could and – not unimportantly – may bring back to life. Those who like these kinds of discoveries are alive at exactly the right time.